In scale-up architecture
A single server is used to serve the QlikView applications. In this case, as more throughput is required, bigger and/or faster hardware (e.g. with more RAM and/or CPU capacity) are added to the same server.
 |
The Scale-up architecture |
In scale-out architecture
More servers are added when more throughput is needed to
achieve the performance necessary. It is common to see the use of commodity servers in
these types of architectures.
As more throughput is required new servers are added, creating
a clustered QlikView environment. In these environments, QlikView Server supports load
sharing of QlikView applications across multiple physical or logical computers.
QlikView load
balancing refers to the ability to distribute the load (i.e. end-user sessions) across the cluster
in accordance with a predefined algorithm for selecting which node should take care of a certain
session. QlikView Server version 11 supports three different load balancing algorithms.
Below is a brief definition of each scheme. Please refer to the QlikView Scalability Overview Technology white paper for further details.
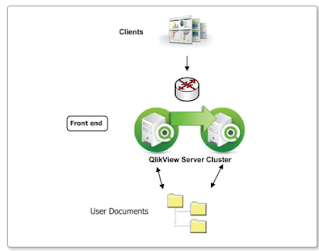 |
The scale-out Architecture |
Random: The default load-balancing scheme. The user is sent to a random server, no matter if the QlikView application the user is looking for is loaded or not on a QlikView Server.
Loaded Document: If only one QlikView Server has the particular QlikView application loaded, the user is sent to that QlikView Server. If more than one QlikView Server or none of the QlikView Servers have the application loaded, the user is sent to the QlikView Server with the largest amount of free RAM.
CPU with RAM Overload: The user is sent to the least busy QlikView Server. Please note that this report does not go into detail on when to use and how to tune different
load balancing algorithms for best performance.
Cluster test executions presented in this report has been run in an environment configured with a better performing scheme for certain conditions of a particular test.
Comments
Post a Comment
Thanks for your message. We will get back you.